ollama下载安装: https://ollama.com/download
根据自己的系统选择下载即可
文章导航
Ollama部署大模型
ollama run qwen:7b
等待部署完成,测试

测试API
curl http://localhost:11434/api/chat -d '{
"model": "qwen:7b",
"messages": [
{
"role": "user",
"content": "你好?"
}
]
}'

测试非流式API
curl http://localhost:11434/api/chat -d '{
"model": "qwen:7b",
"stream": false,
"messages": [
{
"role": "user",
"content": "你好?"
}
]
}'

Ollama自定义Embedding模型
模型转换和定义
上文说到,如何通过微调Embedding模型提升RAG(检索增强生成)在问答中的召回效果 微调后的效果更好
源码拉一下
git clone git@github.com:ollama/ollama.git
cd ollama
然后下载llama.cpp
git submodule init
git submodule update llm/llama.cpp
安装依赖
conda create -n ollama python=3.9 -y
conda activate ollama
pip install -r llm/llama.cpp/requirements.txt
下载模型
export HF_ENDPOINT=https://hf-mirror.com
huggingface-cli download --resume-download moka-ai/m3e-base --local-dir ./moka-ai/m3e-base --local-dir-use-symlinks False
转化模型
python llm/llama.cpp/convert-hf-to-gguf.py moka-ai/m3e-base --outtype f16 --outfile converted.bin
python llm/llama.cpp/convert-hf-to-gguf.py /data1/chenhao/codes/LLaMA-Factory/saves/Qwen2-7B/full/qwen2_v1 --outtype f16 --outfile qwen2-7b-ftconverted16.bin
如果出现

把chkhsh生成的字符串加在llm/llama.cpp/convert-hf-to-gguf.py代码里面

模型生成和使用
Modelfile
FROM converted.bin
TEMPLATE "[INST] {{ .Prompt }} [/INST]"
制作模型
ollama create m3e-base -f Modelfile

使用模型
curl http://localhost:11434/api/embeddings -d '{
"model": "m3e-base",
"prompt": "The sky is blue because of Rayleigh scattering"
}'

如果有GPU,可以看到显卡调用

AnythingLLM安装和使用
下载安装:https://useanything.com/download

配置问答模型


这里有很多模型可以选择,我这里选择llama3-8b,模型会在后台自动下载。
如果你有其他llm相关的模型都可以选择

也可以使用刚刚ollama部署的qwen-7b模型

配置Embedding嵌入模型
这里我们使用微调过的m3e模型, 可以根据自己的需求选择向量数据库,我这里使用默认的数据库


知识问答
点击创建一个workspace,导入知识


配置完成,开始问答


一个简单的本地知识库就搭建成功了,过程中我们没有使用任何第三方需要花钱的模型或api,完全白嫖
补充Linux安装Ollama慢
Linux下安装ollama, 采用官方安装方案时会很慢,可以采用如下方案
sudo curl -L https://xdfe-new.oss-cn-hangzhou.aliyuncs.com/gpt/ikmore/ollama-linux-amd64 -o /usr/bin/ollama
sudo chmod +x /usr/bin/ollama
sudo useradd -r -s /bin/false -m -d /usr/share/ollama ollama
Create a service file in /etc/systemd/system/ollama.service
:
[Unit]
Description=Ollama Service
After=network-online.target
[Service]
ExecStart=/usr/bin/ollama serve
User=ollama
Group=ollama
Restart=always
RestartSec=3
[Install]
WantedBy=default.target
开机启动
sudo systemctl daemon-reload
sudo systemctl enable ollama
# 启动ollama
sudo systemctl start ollama

安装完成
自定义大模型
Modelfile
FROM qwen7b-ftconverted.bin
TEMPLATE """{{ if .System }}<|im_start|>system
{{ .System }}<|im_end|>{{ end }}<|im_start|>user
{{ .Prompt }}<|im_end|>
<|im_start|>assistant"""
PARAMETER stop "<|im_start|>"
PARAMETER stop "<|im_end|>"
延展阅读:
如何利用AI大模型知识库提升电商客服的效率和质量:深度探索检索增强生成(RAG)在客服中的应用
统一知识库多渠道应用:能否通过智能化解决客户服务效率与成本挑战?
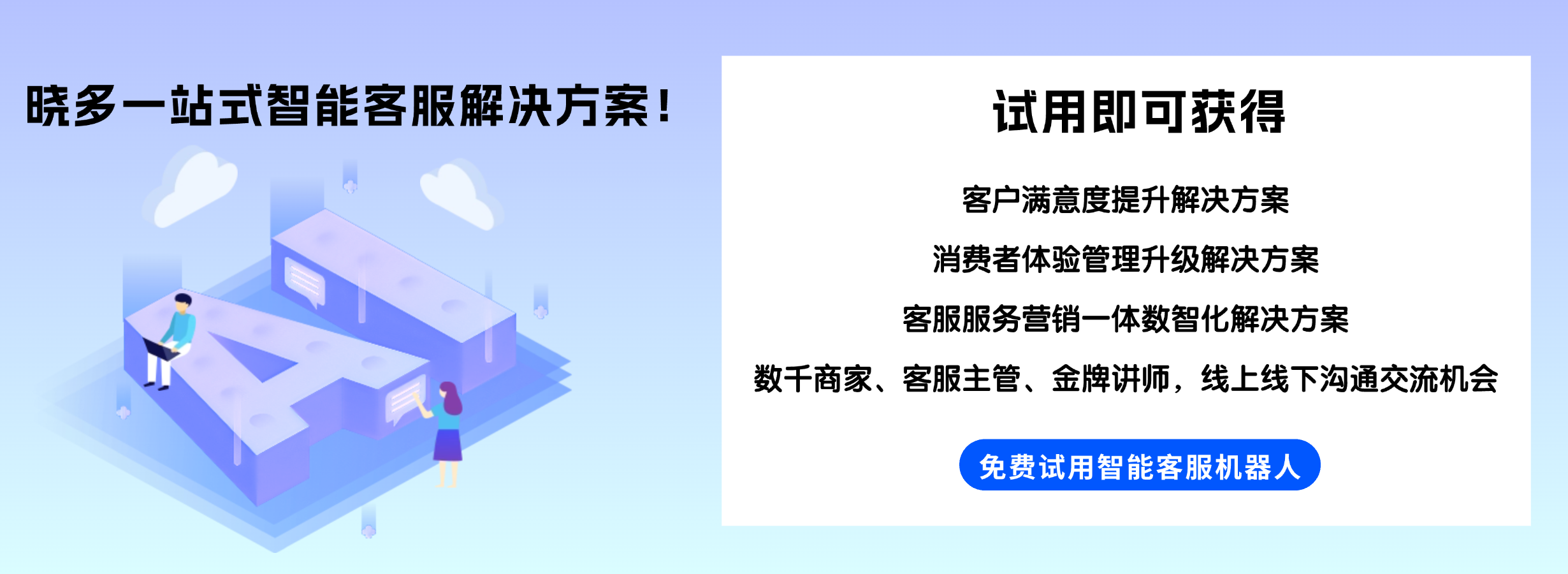