一、创建笔记本

笔记本实例类型就是选择的机器配置大小
二、准备训练环境
alpaca: https://github.com/snowolf/alpaca-on-amazon-sagemaker
vicuna: https://github.com/qingyuan18/finetune-vicuna-on-sagemaker.git
案例中采用了vicuna完成demo训练
需要拷贝这几个文件

训练步骤
安装sagemaker,用于调度算力资源,存储资源等
!pip install -U sagemaker
Clone FastChat 用于训练
!git clone https://github.com/lm-sys/FastChat.git
模型下载

使用s5cmd 加速下载加载模型

制作一个docker镜像用于训练
dockerfile
%%writefile Dockerfile
## You should change below region code to the region you used, here sample is use us-west-2
From 763104351884.dkr.ecr.us-west-2.amazonaws.com/huggingface-pytorch-training:1.13.1-transformers4.26.0-gpu-py39-cu117-ubuntu20.04
ENV LANG=C.UTF-8
ENV PYTHONUNBUFFERED=TRUE
ENV PYTHONDONTWRITEBYTECODE=TRUE
# RUN python3 -m pip install git+https://github.com/huggingface/transformers.git@97a3d16a6941294d7d76d24f36f26617d224278e
RUN pip3 uninstall -y deepspeed && pip3 install deepspeed
## Make all local GPUs visible
ENV NVIDIA_VISIBLE_DEVICES="all"
登陆

推送

编写一个 deepspeed配置 ds.json
%%writefile ds.json
{
"fp16": {
"enabled": true,
"auto_cast": false,
"loss_scale": 0,
"initial_scale_power": 16,
"loss_scale_window": 1000,
"hysteresis": 2,
"min_loss_scale": 1
},
"optimizer": {
"type": "AdamW",
"params": {
"lr": "auto",
"betas": "auto",
"eps": "auto",
"weight_decay": "auto"
}
},
"scheduler": {
"type": "WarmupLR",
"params": {
"warmup_min_lr": "auto",
"warmup_max_lr": "auto",
"warmup_num_steps": "auto"
}
},
"zero_optimization": {
"stage": 3,
"overlap_comm": true,
"contiguous_gradients": true,
"sub_group_size": 1e9,
"reduce_bucket_size": "auto",
"stage3_prefetch_bucket_size": "auto",
"stage3_param_persistence_threshold": "auto",
"stage3_max_live_parameters": 1e9,
"stage3_max_reuse_distance": 1e9,
"stage3_gather_16bit_weights_on_model_save": true
},
"gradient_accumulation_steps": "auto",
"gradient_clipping": "auto",
"steps_per_print": 2000,
"train_batch_size": "auto",
"train_micro_batch_size_per_gpu": "auto",
"wall_clock_breakdown": false
}
根据需求修改覆盖一下train.py

编写训练脚步ds-train.sh

这一步,用Estimator使用docker进行训练,将算力资源与docker镜像进行绑定,并开始训练
https://sagemaker.readthedocs.io/en/stable/api/training/estimators.html

三、训练问题
1、数据集缺失
需要上传数据集到S3,然后打开S3的公共访问通道,通过wget获取数据集


2、训练慢
第一轮训练5个小时了,现在才5%,停止训练,调整了一下参数

第二轮训练,将ds.json中的stage修改为1,修改训练参数如下
deepspeed --num_gpus=8 FastChat/fastchat/train/train_mem.py \
--deepspeed ds.json \
--model_name_or_path "/tmp/llama_pretrain/" \
--data_path selling_point_0517_train.json \
--output_dir "/tmp/llama_out" \
--num_train_epochs 4 \
--per_device_train_batch_size 16 \
--per_device_eval_batch_size 16 \
--gradient_accumulation_steps 16 \
--evaluation_strategy "no" \
--save_strategy "no" \
--save_steps 2000 \
--save_total_limit 1 \
--learning_rate 2e-5 \
--weight_decay 0. \
--warmup_ratio 0.03 \
--lr_scheduler_type "cosine" \
--logging_steps 1 \
--cache_dir '/tmp' \
--model_max_length 2048 \
--gradient_checkpointing True \
--lazy_preprocess True \
--fp16 True \
--tf32 True \
--report_to "none"

速度提升,大概需要34个小时。
3、训练任务自动停止
第二天看进程被杀死,排查是因为创建的训练任务默认只运行24h,24小时后会自动杀死,并且无法重启继续训练。
修改训练参数,添加max_run参数为2天,开始训练

发现24小时后还是被杀死了,在排查是因为这个参数添加错误,应该加在sagemaker的es调度上

开始训练后,终于完成了模型的训练

四、笔记本复现
https://jupyter-docker-stacks.readthedocs.io/en/latest/using/selecting.html
docker run -it -p 10000:8888 \
-v /Users/chenhao/data/jupyter:/home/jovyan \
-e GRANT_SUDO=yes \
-e OPENAI_API_KEY=sk-xx \
--user root \
--restart=always \
--name notebook jupyter/scipy-notebook
http://127.0.0.1:10000/lab?token=359fbdc42b648860d21e1e1e693a85ea53c12a489aa5922a

这样会有一个坑,就是容器中无法使用docker,容器中使用docker需要用到docker:dind这个镜像作为基础镜像,一步一步的安装上来
运行一个容器
docker run --privileged -d -it -p 10001:8888 \
--user root \
--restart=always \
--name docker-in-docker docker:dind
安装依赖
apk add alpine-sdk coreutils dpkg dpkg-dev re2c
apk add python3 py3-pip
apk add build-base python3-dev
apk add libffi-dev python3-dev py3-pip build-base
pip3 install 'jupyter_server>=2.0.0'
pip3 install jupyterlab
启动
mkdir /opt/codes
jupyter lab --allow-root --ip 0.0.0.0 --notebook-dir /opt/codes
打开 http://127.0.0.1:10001/lab/

终端访问docker

安装conda,有个坑,不能选择和系统架构一致版本安装,很奇怪
https://github.com/ContinuumIO/docker-images/blob/master/miniconda3/alpine/Dockerfile
apk add --no-cache bash wget
cp /etc/nsswitch.conf /etc/nsswitch.conf.backup
wget -q -O /etc/apk/keys/sgerrand.rsa.pub https://alpine-pkgs.sgerrand.com/sgerrand.rsa.pub && \
wget https://github.com/sgerrand/alpine-pkg-glibc/releases/download/2.34-r0/glibc-2.34-r0.apk && \
apk add --allow-untrusted glibc-2.34-r0.apk
# Restore nsswitch.conf
mv /etc/nsswitch.conf.backup /etc/nsswitch.conf
apk add -q --no-cache bash procps && \
wget --quiet https://repo.anaconda.com/miniconda/Miniconda3-py310_22.11.1-1-Linux-x86_64.sh -O miniconda.sh
rm -rf /opt/conda
sh miniconda.sh -b -p /opt/conda
ln -s /opt/conda/etc/profile.d/conda.sh /etc/profile.d/conda.sh
echo "source /opt/conda/etc/profile.d/conda.sh" >> ~/.bashrc
echo "conda activate base" >> ~/.bashrc
source /root/.bashrc

如何给jupyter lab 添加python Kernel
- 首先,创建一个新的 Python 虚拟环境。你可以使用 Python 的
venv
模块或conda
来创建虚拟环境。以下是使用venv
创建虚拟环境的命令(我们将新的虚拟环境命名为myenv
): - 激活这个新的虚拟环境:
- 在虚拟环境中安装
ipykernel
,这是 Jupyter 需要的一个库,用于运行 Python 内核: - 使用
ipykernel
将这个新的 Python 环境添加为 Jupyter 内核:
在这个命令中,--name
参数是你想要在 Jupyter 中显示的内核名称。
一个简单的笔记本就完成了模拟了,可以在容器中独立使用docker和conda
体验地址:http://10.248.33.108:8888/lab
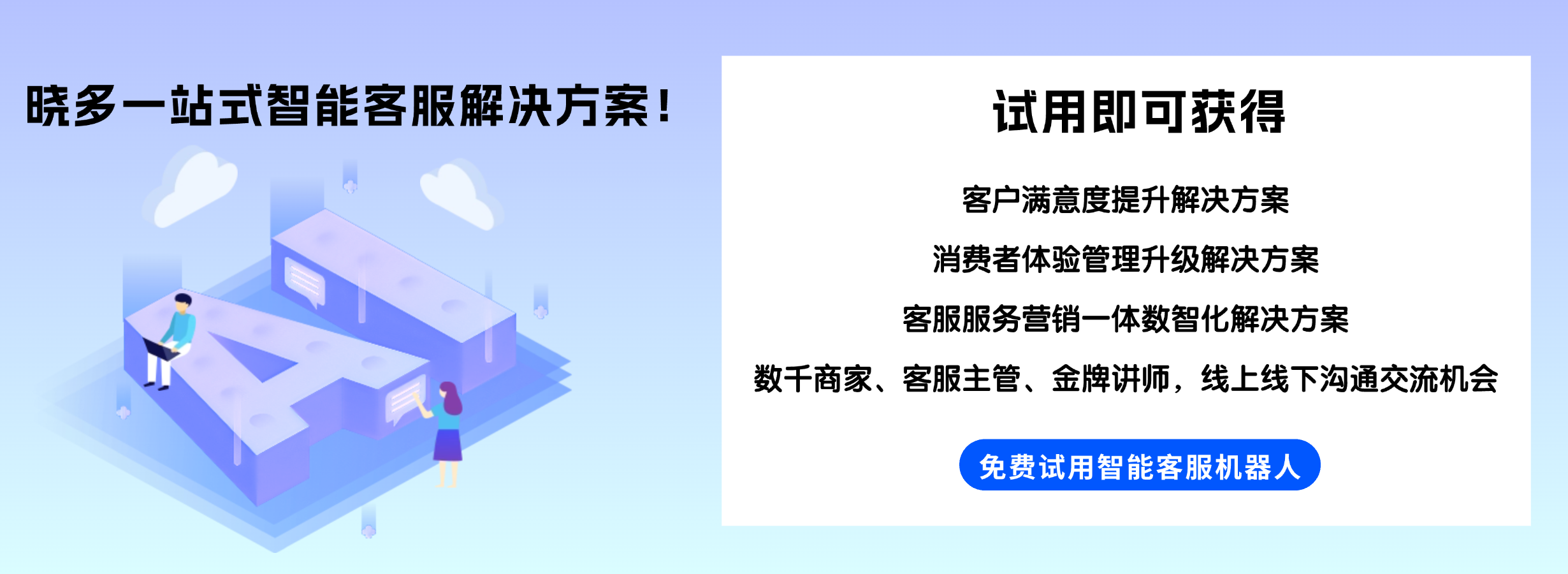